3 Reasons Why Real-Time Analytics Is More Affordable Than You Think
May 17, 2021
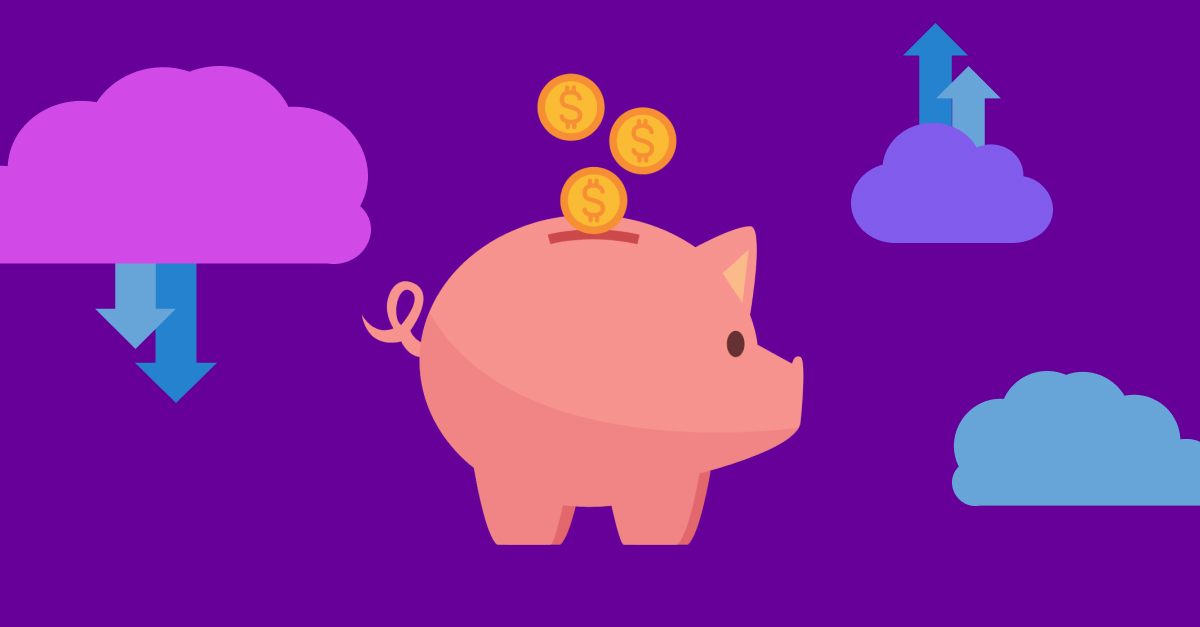
Faster is almost always better in the world in which we live. We cheer when Usain Bolt wins, count on Google Maps to find us the fastest routes and wish Amazon could deliver in hours rather than days. Given the premium placed on speed, real-time analytics—fast queries on data that is seconds and minutes old—can undoubtedly be very valuable to organizations. So what’s preventing them from employing real-time analytics more broadly?
Real-time analytics is often associated with greater cost, and this perception gives engineering teams pause. Sure, fast cars are awesome, but that Ferrari is going to cost a ton. Similarly, engineering teams understand that the ability to analyze and act on real-time data can bring considerable business value. But they may be of the impression that real-time analytics will require significant budget, time or effort and may delay or shelve these projects because of this.
Real-time analytics does not have to be a luxury item though. It does not have to be out of reach for all but the most well-resourced organizations. Advances in technology and the availability of purpose-built products serving this need allow even small start-ups to benefit from real-time analytics today. If you had thought real-time analytics would be useful but too great of an investment in the past, here are some good reasons to reconsider.
There are smarter paths to real-time analytics than simply adding infrastructure
When considering real-time analytics, the first thought is often to add infrastructure to make everything go faster—to improve query latency or to analyze more recent data. For many, this means expensive infrastructure as well, running analytics in-memory to boost speed. But there are more cost-effective ways of achieving real-time analytics than through brute-force methods, so how can we make our infrastructure work smarter?
One way would be to exploit the memory-storage hierarchy more fully to arrive at the right mix of price and performance. Using SSDs where appropriate, instead of relying primarily on in-memory performance, can provide significant cost savings. Taking it a step further, the automated placement of cold data in cheaper cloud storage, while serving fast analytics off hot data in SSDs, can make real-time analytics even more affordable.
Another option is to use more intelligent approaches to data retrieval that tax infrastructure less. Indexing data to accelerate queries is a common strategy here. Indexing generally results in a higher storage requirement but can save much more in terms of compute because queries only have to touch the index rather than scan entire tables. This is a beneficial tradeoff in most instances, as compute is a more expensive resource compared to storage.
Real-time analytics does not have to require a lot more engineering effort
Engineering teams have many questions around the level of effort needed to deliver on real-time analytics, and rightly so. Will more demanding analytics lead to reliability issues on their OLTP systems? Is more data engineering required to build and maintain data pipelines to real-time data sources? Would they be doubling operational complexity by adding a real-time component to an existing batch processing architecture? There are multiple ways to mitigate these concerns and make the real-time analytics effort manageable.
Having separate systems for analytical and transactional workloads is a common design pattern. Using systems optimized for each role, organizations can avoid a lot of performance and reliability engineering that stem from repurposing a single system for both OLTP and real-time analytics. By leveraging existing building blocks, like prebuilt connectors and change data capture (CDC), teams can minimize the data engineering needed to support real-time analytics.
The cloud is also an important ally in reducing operational complexity. Many technologies that are helpful in building out a real-time analytics stack, such as streaming platforms, real-time databases and cloud storage, are offered as-a-Service. PaaS offerings will take the burden of managing infrastructure off engineering teams. For even greater simplicity, SaaS and serverless offerings will abstract away cluster design and capacity planning. With the benefit of cloud services, organizations are able to do more with real-time analytics without growing their teams.
An investment in real-time analytics can be shared across multiple uses
When starting out with real-time analytics, engineering teams are mainly thinking about getting the initial project off the ground. In that context, standing up real-time analytics may appear costly because of the narrow focus on just its first use case, but it would be good policy to weigh its cost against its longer-term potential.
In reality, an investment in real-time analytics has the ability to be leveraged across more applications and more features over time. Organizations will commonly plan to start with an internal application and bring real-time analytics into customer-facing applications thereafter. Others will experience subsequent use cases popping up organically once the initial one is successful. In either case, the architecture and expertise developed for real-time analytics can be shared, and the true cost of real-time analytics should be lower when allocated across these multiple use cases.
Conclusion
Real-time analytics brings organizations considerable value, unlocking revenue, enhancing the customer experience and increasing operational efficiency, but it doesn’t have to be expensive. If you’re looking to maximize your investment in real-time analytics, find out more about Increasing the ROI of Real-Time Analytics.
Image by Free-Photos from Pixabay